06 Jun 2024
|11 min
Thematic analysis
Unlock the secrets of qualitative data with thematic analysis. Learn how to identify patterns and themes from interviews, surveys, and more, to craft compelling narratives.

Welcome to thematic analysis, a puzzler’s dream. Just as a puzzler seeks to fit every piece into place to reveal a complete picture, thematic analysis allows us to piece together seemingly disparate bits of qualitative data to decode and construct the numerous narratives we collect from our subjects.
In this article, I’ll draw on foundational concepts and my practical experience to guide you through the nuances of thematic analysis, its application, and its significance in research. While that may sound a bit dry, thematic analysis is anything but dry.
To start, think of yourself as a puzzle master. Each interview, survey response, or transcript is a unique puzzle. Your task is to assemble the individual puzzle pieces together by identifying patterns and themes that emerge from them. The goal is to craft a “picture” that's both comprehensive and comprehensible.
I’ll break it all down and provide real-world examples from one of my studies. This was a moderated, evaluative study to gather feedback on a new concept using a prototype. The prototype proposed recommendations for optimizing financial performance.
The goals of the study were to evaluate whether users trusted the recommendations, to discover if the analytics that created the recommendations were easy to understand, and to find out if they understood a new financial performance score. I interviewed six participants individually via Zoom.
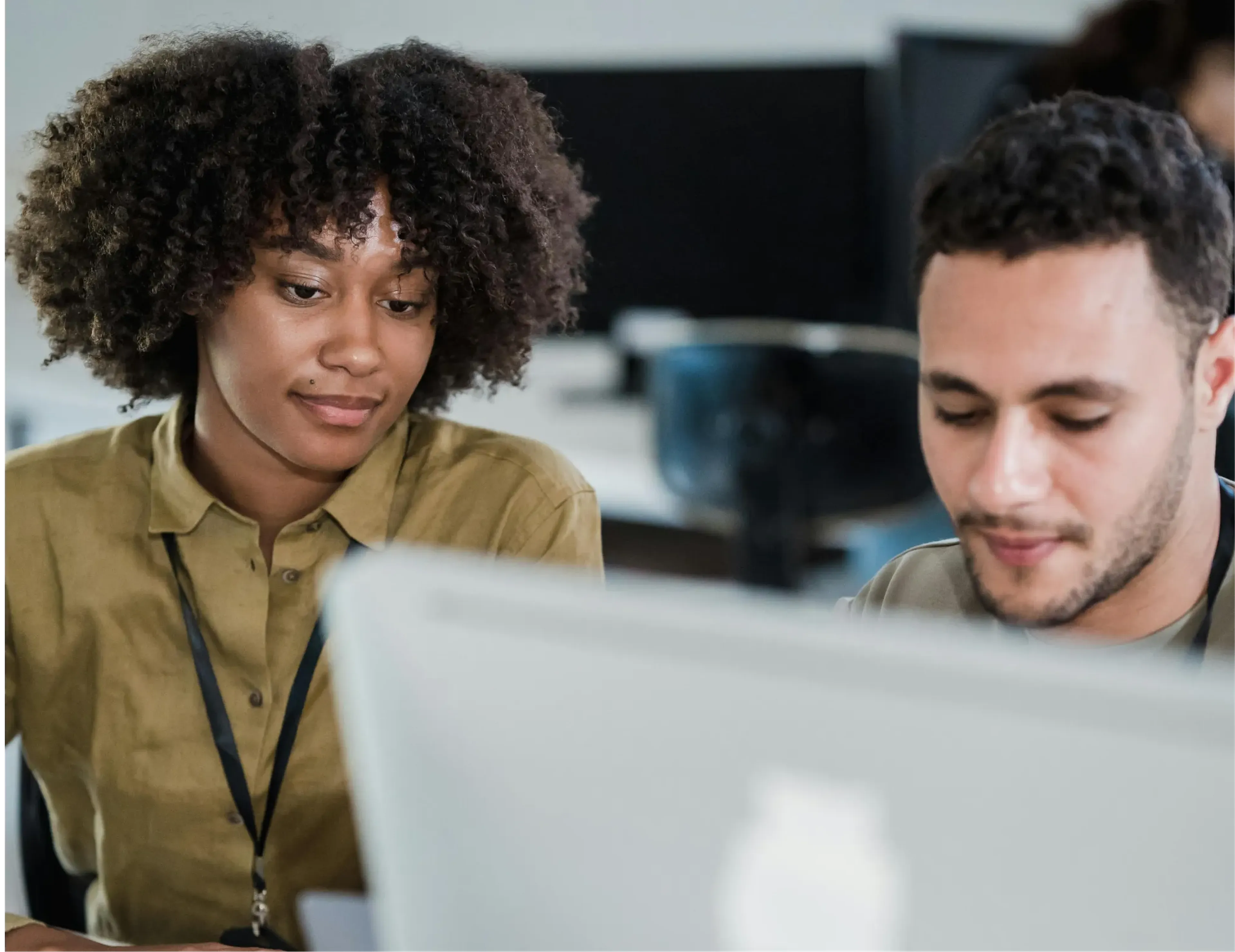
Introduction to thematic analysis
Thematic analysis serves as a technique for pinpointing, examining, and documenting recurring themes in data. At its core, it effectively organizes and details your data collection, but I extend this further to include interpreting different elements around the study topic.
The process of thematic analysis begins with immersing yourself in your data to familiarize yourself with its depth and nuances. This initial step is crucial for identifying significant “snippets” (or aspects or portions) of data that contribute to forming themes.
These themes emerge through a detailed process of coding and categorizing, while reflecting on the core study topics and variances within the data. Thematic analysis is about interpreting your data from multiple viewpoints, to result in a comprehensive understanding of the subject matter.
In my study, themes started to emerge after speaking with the first three participants. First, trust in the financial score and recommendations related to how these calculations were derived. Participants wanted to understand and see the calculations, but the prototype didn't surface this detail.
Second, participants revealed that they typically discuss their optimization efforts with their account managers (AMs). While the recommendations were helpful and appreciated, the relationship with their AM should be taken into primary consideration. The prototype didn't address this potential conflict or synergy between the recommendations and how the participants worked with their AMs.
Elevate your research practice
Join over 320,000+ marketers, designers, researchers, and product leaders who use Lyssna to make data-driven decisions.
What data can be used for thematic analysis?
One of the strengths of thematic analysis is its applicability to a wide range of data. Whether it's semi-structured interviews, diary studies, ethnographies, or surveys, thematic analysis can be applied to understand the underlying themes and patterns. This versatility makes it an invaluable (and very popular!) tool for researchers across various disciplines.
Types of thematic analysis
Thematic analysis can be approached from a bottom-up or top-down perspective. The bottom-up approach is data-driven, allowing themes to emerge organically from the data without preconceived notions. Conversely, the top-down approach can be more theory-driven, where you look for specific themes based on existing theories or research objectives.
In my study, the two themes I mentioned above were substantiated in subsequent sessions. Let's call them “Trust” and “AM Role.” The team and I had no discussions or assumptions about the role of AMs, so the “AM Role” theme is an example of a bottom-up approach.
We did have the assumption that participants would scrutinize the score and want detailed information about how it was calculated. The theme “Trust” is an example of the top-down approach. I was looking for feedback on this aspect specifically.
I coded or categorized data according to each separate assumption, whether they were validated or not.
When to use thematic analysis
Thematic analysis is particularly useful when dealing with complex data sets where you want to understand broad patterns and narratives.
It's an extremely flexible method (like Gumby flexible), making it super suitable for exploratory studies where the research questions may have evolved. It’s also ideal for some evaluative, moderated studies, such as the example above.
Thematic analysis vs content analysis
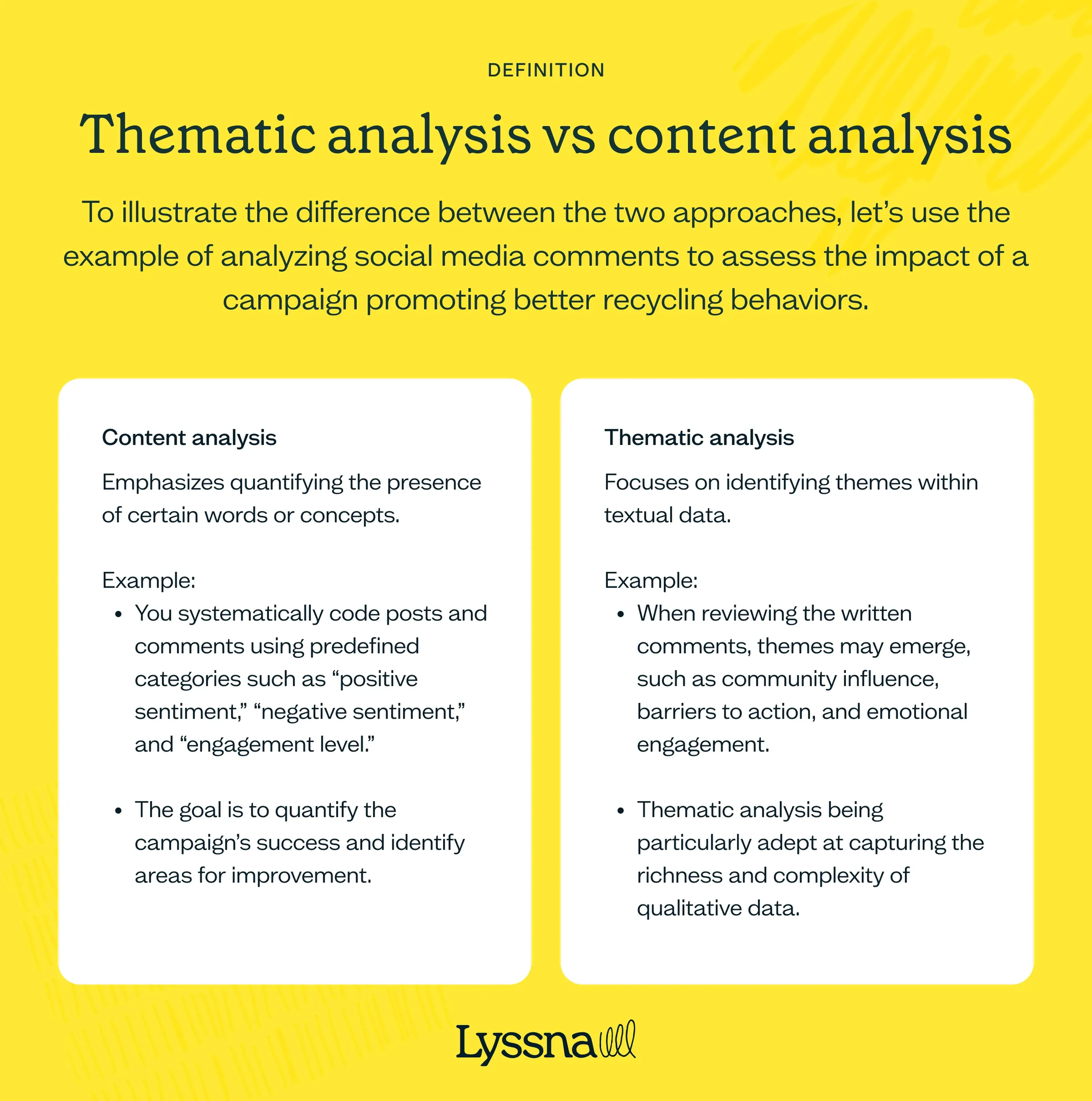
While thematic analysis focuses on identifying themes within textual data, other methods like content analysis might emphasize quantifying the presence of certain words or concepts.
To illustrate the difference between the two approaches, let’s use the example of analyzing social media comments to assess the impact of a campaign promoting better recycling behaviors.
Content analysis: You systematically code posts and comments using predefined categories such as “positive sentiment,” “negative sentiment,” and “engagement level.” The goal is to quantify the campaign’s success and identify areas for improvement.
Thematic analysis: When reviewing the written comments, themes may emerge, such as community influence, barriers to action, and emotional engagement.
Each method has its unique focus and application, with thematic analysis being particularly adept at capturing the richness and complexity of qualitative data.
How to do thematic analysis
Below is a step-by-step summary of how to conduct a thematic analysis.

1. Prepare the data
Start by organizing your data in a manageable form.
I set up my notes in spreadsheets, organized by question, topic, and assumption. These are delineated with separate lines and color codes.
If I haven’t done this in advance, I repurpose my notes or transcripts into a spreadsheet, using this same approach, and break apart “snippets” from participants verbatim into smaller, more manageable pieces.
2. Familiarize yourself with the data
Immerse yourself in the data by reading and re-reading the content, and writing down initial themes (or categorization) ideas.
Re-familiarize yourself with your research plan before starting this phase to make sure your research goals are top of mind.
3. Code the data
Begin coding your data, tagging segments (aka “snippets”) with codes that summarize or capture the essence of that segment.
Start with short, direct theme names, such as “Trust” or “Opportunities.”
4. Develop themes
Continue to apply codes to potential themes, gathering all data relevant to each potential theme.
Briefly define your theme names and add a key or legend to your working document. For example, you could expand on the “Trust” theme with context, such as “Trust in score and recommendations relate to transparency.” Adding a short description with context will help you remember where you started (because the theme will likely evolve throughout the process) and also help your stakeholders understand what the theme means in relation to the study overall.
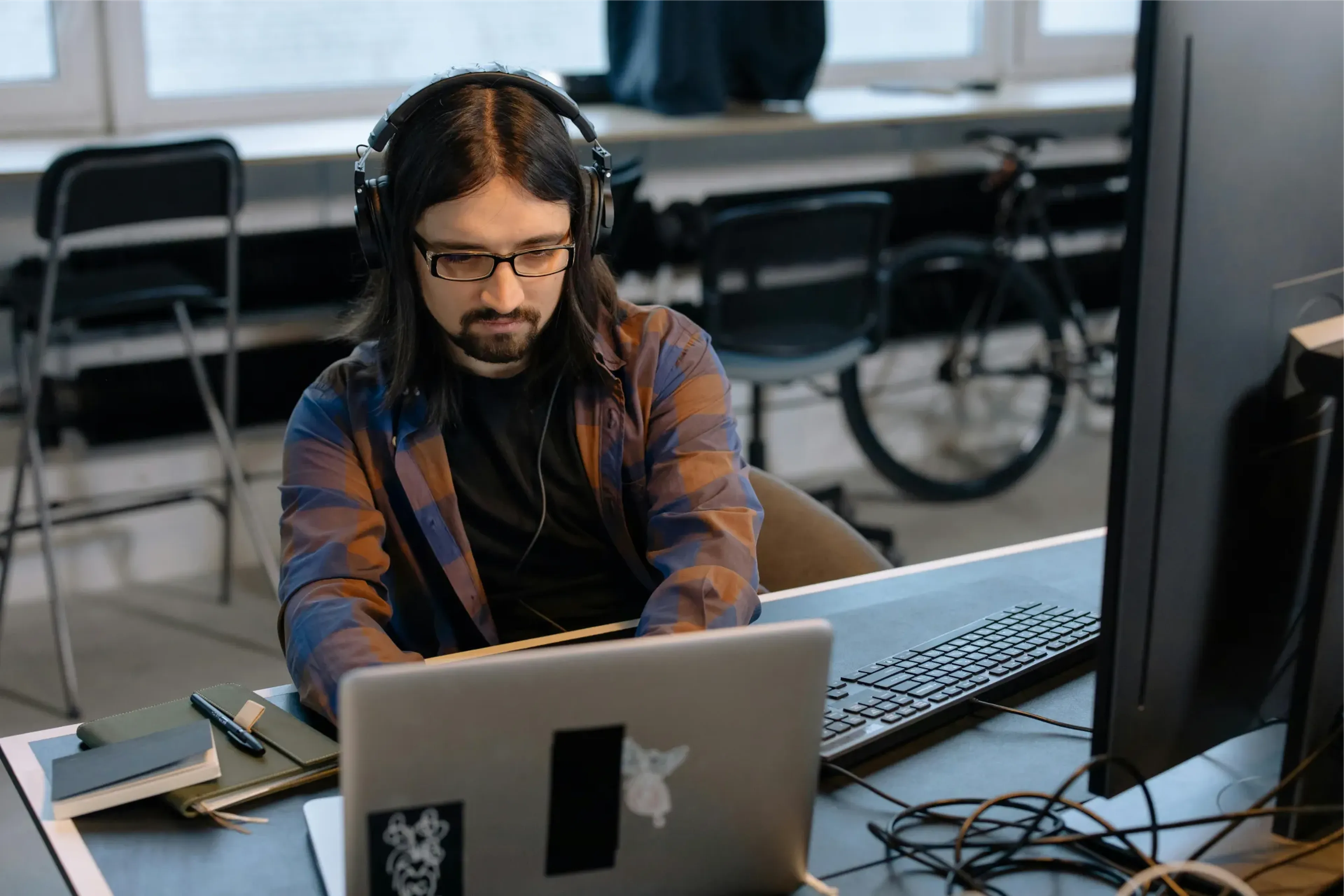
5. Review themes
Check if the themes work in relation to the coded extracts and a large portion of the dataset.
Not all themes are equally important. Some themes will appear in quantity, but aren't quality themes. Other themes may not be actionable. And not all snippets will fit nicely into one thematic code set. That’s common. When outliers appear, place them in a theme called “Miscellaneous” or “Other.”
Repeat your theme identification and coding process at least three times. I often use my frameworks tool for inspiration. The tool inspires me to think of various “lenses” or “perspectives” to synthesize my data, and forces me to look at the datasets in interesting and unique ways.
6. Define and name themes
Refine the specifics of each theme and determine what aspect of the data each theme captures.
Revisit your theme names regularly as you progress, and update them as you see fit. Once you get comfortable with this process, you’ll likely be able to identify sub-themes in several categories. In our example, “Trust in score and recommendations relate to transparency,” there are sub-themes as to what calculation details participants want to see and where they want to see them.
Sub-themes may be practical, logistical, realistic, behavioral, attitudinal, or otherwise, and can be categorized as such.
7. Write up your analysis
Your analysis should relate to the research questions and be discussed with your stakeholders. Ultimately, you should strive to provide a narrative that ties together the themes and offers insights into the data.
Whenever possible, include your stakeholders in this process. I typically craft 5–6 short themes with bullet-point descriptions for my team to review, and I ask them for feedback before progressing. I’m particularly interested in which themes resonate the most for them and why, what themes they think are most actionable, and if they have any themes to add. This dialogue is paramount for focusing my remaining efforts in the most productive way possible.
What my stakeholders see as pertinent themes and what I see as pertinent themes will likely differ. Discuss the various perspectives and allow this dialogue to inform your takeaways, narratives, and reports.
Your go-to user research platform
The best teams use Lyssna so they can deeply understand their audience and move in the right direction — faster.
Mastering thematic analysis: Unlocking the power of qualitative data
Thematic analysis serves as a bridge between data collection and discovering the underlying messages within. By following a structured process, you can identify deeper meaning, contributing to a more well-rounded and expansive understanding of your research topic.
This method's flexibility and accessibility make it a top choice among qualitative researchers, especially those who prefer working with text-based tools such as Google Docs or spreadsheets, to uncover narratives within complex data sets.
--
This article was authored by Michele Ronsen, Founder and CEO of Curiosity Tank. Michele is a user research executive, coach and educator. She teaches design and user research to people around the world. Her corporate trainings and workshops are inspired by working with Fortune 500s and start-ups for more than twenty years. Fuel Your Curiosity is her award-winning, free, user-research newsletter. In 2020, LinkedIn honored Michele with a TopVoices award in the Technology category. She is the first and only researcher to receive this award.
You may also like these articles
Try for free today
Join over 320,000+ marketers, designers, researchers, and product leaders who use Lyssna to make data-driven decisions.
No credit card required